The Inside series is a column where the Tech in Asia Jobs team gives an insider’s glimpse into interesting companies and professions. Grab is now hiring on Tech in Asia Jobs.
While many are familiar with ride-hailing app Grab, few of us really know what happens after we hit “book.”
Different teams work the magic to deliver the ride, but I was interested in how data science fits into all of this. So I sat down with Lye Kong-wei, Grab’s head of data science, to find out more.
Making sense of the data Grab collects
Grab hails from Malaysia, starting off as MyTeksi in 2012. In six short years, it has grown into a billion-dollar startup and a top contender in Southeast Asia’s private car-hailing space.
Around 3.5 million rides are booked on the app daily, generating over 10 terabytes of data on the platform each day. More than 60 employees work in the data team in Singapore to make sense of the data and use insights gathered to improve the Grab experience.
The team is expected to expand by 50 percent at the end of 2018.
The data team at Grab
The data team at Grab is divided into two: the data engineering team and the data science team.
The data engineering team manages Grab’s data warehouses, builds its pipelines, and ensures that other data teams get data in a form they can readily use.
Headed by Lye, the data science team is made up mostly of researchers working on models and algorithms to translate research into product features.
“From the moment a passenger opens the Grab app to the time a vehicle arrives, data science powers the thinking and decision-making on the most efficient routes, travel time, and price point. These collectively work to make a safe and convenient commuting experience for both drivers and passengers,” says Lye.
There are around 30 people in the data science team. It’s currently based in Singapore, but there are plans to expand to other countries where Grab operates.
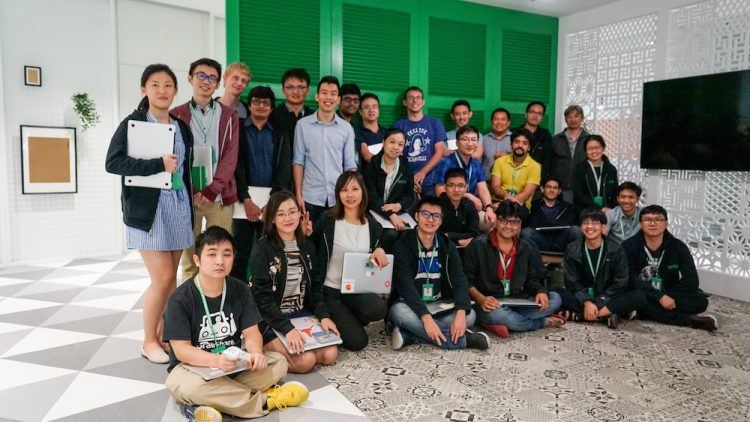
Grab’s data science team. Lye is at the back, on the far right. Photo credit: Grab
Team structure and dynamics
Grab’s data science team is made up of five groups focused on specific areas.
1. Machine learning
The machine learning team works on all kinds of predictions using traditional machine learning and new deep learning techniques. Most applications involve studying users’ behaviour to improve the experience for both passengers and drivers.
2. Markets
Working closely with the machine learning team, the markets team studies supply (driver) and demand (passengers). They are responsible for matching drivers and passengers by forecasting fixed fares amid price fluctuations. Their driver booking system is an example of this.
“We have learned our drivers’ preferences and behaviours, enabling us to predict which jobs drivers will take,” explains Lye. “For instance, many GrabBike drivers in Jakarta have a ‘home base’ which they prefer not to veer too far from, no matter how profitable a ride might be. Bookings are then sent to drivers with the highest probable booking rate. Because of this, our drivers now receive jobs they prefer and get better earning opportunities.”
3. Optimization
By developing and managing services like GrabHitch, GrabShare, and GrabShuttle, the optimization team helps put more people in fewer cars and make cities less congested.
“This team also forms the backbone of our collaboration with governments, which use travel and traffic data to improve transport and city planning,” notes Lye.
4. Simulation
The simulation team helps Grab’s country teams simulate how passengers and drivers would interact with new services and respond to tweaks in existing ones. The team constantly improves their services as a result of these simulations.
5. Architecture
Looking after the lower layers of the stack, the architecture team works mostly on experimenting and rapidly adopting new technologies to increase the speed at which Grab innovates. For example, it has used GPUs (graphics processing units) to reduce the data team’s processing times for even faster real-time insights.
Case study
A significant project the data science team is working on is GrabShare, Grab’s commercial service that enables passengers to carpool with another passenger heading in the same direction.
“To get passengers quickly to their destinations, GrabShare pairs just two passenger bookings with similar trip routes within a single trip,” says Lye.
Passengers will experience a maximum of two stops before reaching their destinations.
GrabShare focuses on maximizing drivers’ potential earnings by reducing the time and distance spent on a single GrabShare ride, allowing drivers to complete more jobs per hour to boost their income and reduce fuel consumption.
Two key metrics are involved in doing this:
- Match rate – This measures how well they match the first passenger with another passenger going in the same direction.
- Match quality – This measures the trade-off in time a passenger faces by choosing to share a ride with someone else.
The key is to strike a balance between match rate and match quality, while aiming for higher efficiency in putting more people in fewer cars.
“With this, it’s important to understand how passenger behavior differs from one market to another,” says Lye. “For example, GrabShare riders in Singapore are less willing to wait for a ride than GrabShare riders in Indonesia.”
GrabShare’s history
- The first version of the GrabShare algorithm was developed in 2015 when Grab launched their GrabHitch service. GrabHitch is GrabShare’s non-commercial ride-sharing counterpart.
- Once users got more familiar with ride-sharing on GrabHitch, the data science team started studying data related to driver and passenger behavior.
- The team then simulated the GrabShare user experience for drivers and passengers, and refined its features.
- GrabShare launched in December 2016.
- The teams then spent time on the ground to tweak the product for the next few markets before launching GrabShare in those markets.
“The GrabShare algorithm continuously evolves as every ride on our platform is logged, analyzed, and adjusted according to the local needs of each city,” says Lye.
Challenges
Communication
Lye says that it can sometimes be hard to explain their work to their colleagues outside the data science team, both in terms of its impact on the business and the opportunities it offers.
To address this, his team has started holding data science talks for all Grab personnel, highlighting specific projects and areas of focus for the data science team.
Hiring
Finding great data scientists at the volume that Grab needs is also a challenge.
One way the team deals with this is by engaging in more external activities, such as encouraging its data scientists to network, speak in technical forums, attend relevant courses, as well as to blog or publish their work.
Hiring data scientists at Grab
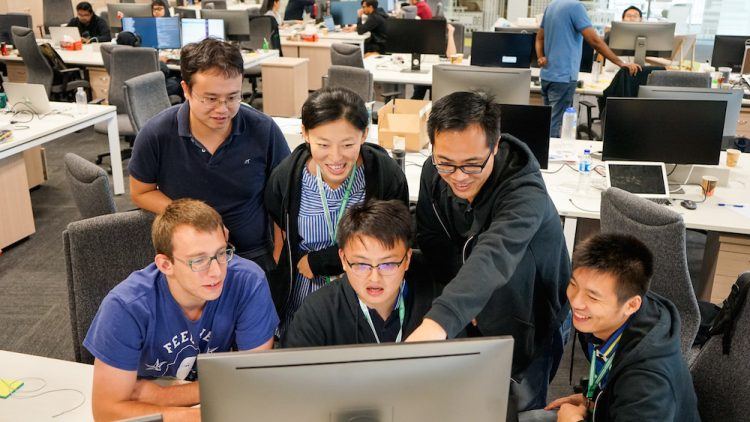
Grab data scientists in a discussion. Photo credit: Grab
Candidates are first screened for the basics, such as communication skills. They then typically go through three rounds of interviews.
The first round is with one or more of their peers, where candidates are assessed for their technical capabilities. They look out for good theoretical fundamentals, as well as relevant working or personal experience.
The next round is with the hiring manager, who evaluates if candidates are fit for the role in terms of potential performance and culture.
The final round is with the head of the data science department.
In addition, Lye says they look out for what they call the “hidden diamond” in every candidate: character.
“A diamond needs extreme heat, time, and pressure to be made,” he observes. “Similarly, character takes years to form. Integrity, tenacity, and humility are traits we try to elicit from the candidate’s personal stories.”
Lye also leaves potential candidates with some advice.
“Know your destination,” he recommends. “If it is on our way, hop on and share the ride.”
This post How Grab runs its data science team appeared first on Tech in Asia.
from Tech in Asia https://www.techinasia.com/grab-runs-data-science-team
via IFTTT
No comments:
Post a Comment